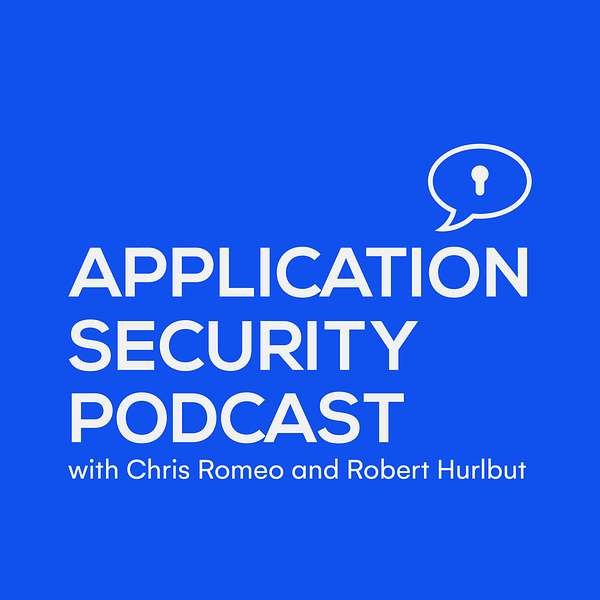
The Application Security Podcast
Chris Romeo and Robert Hurlbut dig into the tips, tricks, projects, and tactics that make various application security professionals successful. They cover all facets of application security, from threat modeling and OWASP to DevOps+security and security champions. They approach these stories in an educational light, explaining the details in a way those new to the discipline can understand. Chris Romeo is the CEO of Devici and a General Partner at Kerr Ventures, and Robert Hurlbut is a Principal Application Security Architect focused on Threat Modeling at Aquia.
The Application Security Podcast
Katharina Koerner -- Security as Responsible AI
Dr. Katharina Koerner, a renowned advisor and community builder with expertise in privacy by design and responsible AI, joins Chris and Robert to delve into the intricacies of responsible AI in this episode of the Application Security Podcast. She explores how security intersects with AI, discusses the ethical implications of AI's integration into daily life, and emphasizes the importance of educating ourselves about AI risk management frameworks. She also highlights the crucial role of AI security engineers, the ethical debates around using AI in education, and the significance of international AI governance. This discussion is a deep dive into AI, privacy, security, and ethics, offering valuable insights for tech professionals, policymakers, and individuals.
Links:
- UNESCO Recommendation on the Ethics of Artificial Intelligence: https://www.unesco.org/en/artificial-intelligence?hub=32618
- OECD AI Principles: https://oecd.ai/en/ai-principles
- White House Blueprint for an AI Bill of Rights: https://www.whitehouse.gov/ostp/ai-bill-of-rights/
- NIST AI Risk Management Framework: https://www.nist.gov/itl/ai-risk-management-framework
- NIST Adversarial Machine Learning: A Taxonomy and Terminology of Attacks and Mitigations: https://csrc.nist.gov/pubs/ai/100/2/e2023/ipd
- Microsoft Responsible AI Standard, v2: https://www.microsoft.com/en-us/ai/principles-and-approach
- ==> Microsoft Failure Modes in Machine Learning: https://learn.microsoft.com/en-us/security/engineering/failure-modes-in-machine-learning
- ENISA Securing Machine Learning Algorithms: https://www.enisa.europa.eu/publications/securing-machine-learning-algorithms
- Google Secure AI Framework (SAIF): https://developers.google.com/machine-learning/resources/saif
- ==> Google Why Red Teams Play a Central Role in Helping Organizations Secure AI Systems: https://services.google.com/fh/files/blogs/google_ai_red_team_digital_final.pdf
Recommended Book:
- The Ethical Algorithm: The Science of Socially Aware Algorithm Design by Michael Kearns and Aaron Roth: https://global.oup.com/academic/product/the-ethical-algorithm-9780190948207?cc=us&lang=en&
FOLLOW OUR SOCIAL MEDIA:
➜Twitter: @AppSecPodcast
➜LinkedIn: The Application Security Podcast
➜YouTube: https://www.youtube.com/@ApplicationSecurityPodcast
Thanks for Listening!
~~~~~~~~~~~~~~~~~~~~~~~~~~~~~~~~~~
We're honored to host Dr. Katarina Koerner, a distinguished senior manager, advisor, and community builder with deep expertise in privacy by design, privacy enhancing technologies, and responsible AI. With an impressive background that spans senior management, legal expertise, and technical acumen, Katarina seamlessly bridges the worlds of business, ethics, privacy, law, and technology. We'll explore the nuances of responsible AI, delve into the intricate relationship between security and AI, and uncover the ethical implications of AI's integration into our lives. From understanding the core principles of responsible AI, to envisioning the future of AI security, get ready for a deep dive into the world of AI with Dr. Katarina Koerner. Hey folks, welcome to another episode of the Application Security Podcast. This is Chris Romeo. I'm the CEO of Devici and a general partner at Kerr Ventures. Happy to be joined today by my good friend Robert Hurlbut. Hey Robert.
Robert Hurlbut:Hey, Chris. Yeah, Robert Hurlbut, and I'm a principal application security architect, as well as threat modeling lead at Aquia, and really glad to be here, uh, talking with our guests and also focused on AI again. Hi,
Chris Romeo:Yeah, it's been a popular topic for us recently. Like we've spent a lot of time talking about AI, but it's, it's a hot topic in. The entire, like everybody's talking about AI, like now, my mom has not yet started using chat GPT, but she's gonna listen to this and probably ask me what that actually means, but okay. We gotta, we gotta hear our guest, Katharina Koerner, her security origin story. So Katharina, if you could just, just tell us how you got into the world of security, however much depth you want to go into, we'd love to hear people's stories.
Katharina Koerner:Thank you so much. Thanks for inviting me. It's an honor to be here. So I moved to Silicon Valley three years ago. And before I moved, I was working as the CEO of a company in the educational field and before that in the public sector in Austria, and I moved for private reasons. My background is a PhD in law. And I was like, what would I work in Silicon Valley? And I was looking at so many job posts and the job market. And I saw that there is a need for people in the field of information security. My brother was like, Oh my God, because he's an InfoSec person. He's passionate about the field. And usually I always, you know, handed him my phone. Could you please, I don't know, install email on my phone? And he's like. So, but I was determined to learn. So, uh, I started with, um, information security management course at the, at University of Krems in Austria, um, pretty high level, security management, business models, IT strategy and stuff like that. And I did this ISO 27001, um, some, some courses on that. I found it super cool, super. Impactful, super important. So structured, I love structure. And then when I had arrived here, I couldn't work for a year because I was waiting for an employment authorization. So I also did this, um, Stanford Advanced Cybersecurity Certificate, um, primarily taught by, um, Dan Boneh, um, where I had to write on every single word to pass the tests because it was all about, you know, using cryptography correctly and writing secure code and, but you know, what I really understood is that this is a vast field with so much subject matter expertise. I also did this SANS security awareness professional, um, that was more like, you know, right up my alley. But in the end I thought actually I cannot catch up with you guys. And, um, so then I tried to combine my legal expertise. In privacy with, um, security and tech and a dove into privacy enhancing technology. So that's something where I really, really tried to become an expert. So I'm really honored to be here today, um, with that background of mine. Thank you.
Chris Romeo:Yeah, well, we're honored to have you as somebody with, with, uh, such a different experience from where we're coming from. But that's, that's what makes these types of conversations so interesting is because you, you have studied so many different things and know so many other things that, uh, Robert and I are both more from the, maybe from the tech side of, of kind of growing up in security from that perspective. Um, and I think this is going to be fascinating because we've got a topic here that I can honestly say. I don't think I even know what a responsible AI principle is. I don't even think I know what those three words together actually mean. Um, and so I'm, I'd love for you to start there, like, build a foundation for us and for our audience as we make our way up to then, talking about how security plays in here. But help us to understand responsible AI principle. What does this, what does this even mean? So
Katharina Koerner:Yeah, happy to. So, um, maybe I start with how I came to the responsible AI ecosystem or the topic. So my last role was, uh, the principal researcher at the International Association of Privacy Professionals. And in that role, I was responsible for privacy engineering. So apart from that, I was a lot on LinkedIn, you know, learning a lot, uh, networking a lot. And I, about two and a half years ago, I stumbled more or less, uh, over responsible, ethical, trustworthy AI. And it was like, wow, that's a huge, you know, field. That's a huge, uh, ecosystem. Just so many people so engaged. How does this relate to privacy? So more or less, um, kind of, you know, the same, um, place where we're coming from today with security. Um, and then I discovered that. There's so much overlap between privacy and responsible AI. And at the beginning, it seemed a fluffy term, responsible AI, responsible AI principles, ethically AI, trustworthy AI. But by now I have an overview and I'm so happy to share that. So it can seem that, so for me, first of all, ethically AI, trustworthy AI, responsible AI, it's, it's all the same. So it's three different terms for one and the same thing. And it seems it's kind of a fluffy thing, but actually it's not anymore. So Responsible AI has quite a clear profile by now. And Responsible AI is a set of good governance principles that are composed of a specific set of common principles that usually include security, privacy, data governance in general, accountability, auditability, transparency, explainability, um, fairness and non discrimination and human oversight or promotion of human values. So there are so many various sources for those Responsible AI principles by now, but they actually do all overlap in those principles that I named in various shapes and forms. Definitely big, huge overlap. So some of those responsible AI principles or this set of guidelines were published by public organizations. We have, for example, the UNESCO's recommendation on ethical AI. We have the Council of Europe published something. We have the OECD, uh, AI, uh, Principles or something published by the European commission. We have nation states that have published, uh, guidelines, ethical, ethical guidelines for the use of AI by China, for example, or in the U S it's the White House Blueprint for an AI Bill of Rights. It's also the same kind of principles. And then we have industry initiatives such as partnership on AI or the global partnership for AI. Same thing, and then we have almost countless, uh, self regulatory initiatives by companies, such as, uh, very good to have a look at because it's really good documents, uh, by Microsoft's Responsible AI Standard. We have Google's Responsible AI Practices, Salesforce, Trusted AI Principles, Facebook's five pillars of Responsible AI, and so on and so forth. And on top of that, we also have standardization body set. Such as ISO, IEC, IEEE, and NIST, which also offer guidance. So, like I said, most of those AI governance frameworks overlap in the definition of principles and security is always one of those principles that is emphasized by those many, many types of organizations.
Chris Romeo:if we kind of think about all of these different types of... Uh, Principles and things that different governments are putting together and, and whatnot. What would be kind of the, the way we would summarize what all of these different groups are trying to do? Is it to ensure that humanity is protected in this AI context or is there, is there some other kind of summation of what everybody's trying to capture across all these different regulations and standards?
Katharina Koerner:Yeah, I think that's exact you, you hit the nail on its head. So it's, I think the common goal is to prevent harm for individuals. Organizations and society at large. or even the whole planet. So one example is a NIST's AI Risk Management Framework, which NIST published in January this year, the first version. And it definitely includes all of those, um, various stakeholders. So even, you know, the planet at large, societies, groups, uh, individuals. So everyone should be protected, um, by a thorough risk management of AI systems.
Robert Hurlbut:So you mentioned NIST and, and some of the work that they've been doing. Uh, what are some other requirements, uh, that. You know, of, of, of responsible AI principle, uh, beyond maybe just NIST, but some other things that you, that you could talk about,
Katharina Koerner:So if we talk about security, do you want to talk about security in particular or about AI risk management in general?
Robert Hurlbut:um, either one, you know, uh, let's talk about the first one. Yeah.
Katharina Koerner:Security? I did some research on what security as a responsible AI principle means and what resources are out there that could explain it a little bit better. And I came across three really good resources. So, for example, um, so first of all, I mean, of course, the number of research papers. Discussing how machine learning can fail, either due to attacks or just, you know, um, without any malicious intention. There are so many of them and it's really hard to keep track. So I think not only engineers. Practitioners, but also lawyers and policy makers need to be aware of security risks because it's really, I think, at the core of those responsible AI principles is security, especially because security is related to so many of the other principles. Privacy, there is no privacy without security, but there is security without privacy, because privacy is, um, dependent on security, uh, measures and good security, right? So protecting personal data is just one of the things that we protect with security, but it's so hard to stay updated on those various threats and defenses, um, related to machine learning systems. So, um, I came across, and by the way, comments are due until end of this month. So in March this year, NIST has It's published an initial draft of its publication Adversarial Machine Learning: A Taxonomy and Terminology of Attacks and Mitigations. Um, it covers All various, uh, you know, attacks that can happen during the life cycle of AI systems, attackers, goals, capabilities, uh, and also goes into the specifics of attacks and mitigations. I can really recommend that. I think it looks to me as if it's a really good, um, document and those, um, attacks range from. I don't know, poisoning attacks, I mentioned regularly, right? Also in the news, uh, it also includes privacy attacks, by the way. So data reconstruction attacks, memorization or membership inference, uh, up to, uh, evasion attacks, uh, white box evasion attacks, black box evasion attacks, and so on. And NIST also highlights and acknowledges that those are really open challenges in AI system security and adversarial machine learning that it's so hard to even detect when a machine learning model is under attack. So this is the first challenge and, or detecting out of distribution inputs that may not confirm to the data distribution the model was trained on. It also acknowledges the challenge for designing effective mitigations, uh, against attacks, which is like, you know, kind of. What it is all about, isn't it? Um, and, uh, lists further challenges. What I find a little bit more accessible already is something else, um, that was published by, um, several people also working, uh, for Microsoft. It's called Adversarial Machine Learning Threat Taxonomy, um, with the title Fail, Failure Modes in Machine Learning. Uh, it's described in detail on Microsoft security blog and. Um, it's a taxonomy actually that came out of Microsoft's, uh, Ether engineering, uh, practices and Ether is part of the responsible AI, um, governance at Microsoft. It's the engineering side of things. Um, so it's definitely spot on, on responsible AI. It's part of their responsible AI governance and it also, um. emphasizes that current threat modeling practices in software development lifecycle needs to need to be, um, updated or, or, you know, extended to identify and address threats in the context of AI and machine learning. They, um, made this, um, threat taxonomy, especially for products and services that interact with AI and machine learning based services and for products and services that, um, are fundamentally built using AI or machine learning. I think this is also a resource that is really accessible because it also has a long, long, long, long list of concrete questions that you can ask in a security review of AI and machine learning. So maybe we have the chance to post it in, in some kind of chat or under the video later. And lastly, I would like to mention ENISA's work. Um, so the European Union Agency for Cybersecurity, they already published, um, uh, um, a report on Securing Machine Learning Algorithms in December, 2021. And it also provides another high level machine learning taxonomy, uh, for identifying cybersecurity threats to machine learning algorithms and their vulnerabilities and what is Hey, What's super concrete about this report is that it lists, uh, various security controls that address these vulnerabilities. And they also took, uh, took into account ISO 27001 or two, or the family, and then NIST, uh, 853 framework. So it's kind of a cross crosswalk also, uh, and all those controls are then linked to their machine learning taxonomy. So I think all of these really. Are really great resources to dig into and to be aware of and are at the core of what security in the context of ai, uh, equal equaling responsible ai, uh, mean. And, uh, I would, I I, I would recommend taking a look at those.
Chris Romeo:How about privacy? So I'm curious about, uh, you, you had mentioned that, um, you know, you can't have, uh, security without privacy. Totally agree. 100%. When we think about privacy at the intersection with AI. What are some of the challenges there? What are some of the things we need to be thinking about and, and I don't want to say worrying about, but what are the, what are, what are some of the potential challenges when we think about AI having access to data and having access to personally identifiable information or personal health information or whatever, whatever types of data it might be, um, as somebody that's studied privacy and has been working in the privacy side, what are, what are things that come to your mind when you think about privacy and AI? Okay.
Katharina Koerner:Yes, of course. So anytime we have, uh, a machine AI or machine learning processing personal data, we're in the realm of privacy. And of course, ai. Machine learning poses complex privacy risks to individuals, but also organizations and society at large or groups, subgroups, um, and just mentioning again, again, that one of the principles of responsibility, AI regularly mentioned, Explicitly refers to privacy, and that is kind of a reminder that the obligation to apply general privacy principles, which, which are the backbone of privacy and data protection laws globally. They also apply to AI and machine learning systems, of course. I think this is not, um, cannot be emphasized often enough because sometimes there's the impression that AI and machine learning is not regulated. already or there are no laws and that's not true because All privacy laws apply to machine learning when personal data is involved. So that in, in imply, um, that implies ensuring, uh, collection limitations with data minimization, big challenge in machine learning, um, data quality, purpose specification. So for which purpose do I collect the data or use the data? I cannot just change the purpose for whatever reason, you know, secondary use you collected for, I don't know, for some. Sending someone some ads about gaming and then you send them something else or use limitation that's related to it and you have to have accountability and individual participation so that there's a choice and that you have a transparent and informed decision making by the end user. And those principles of trustworth you're responsible I like Like I said, just mentioned transparency, for example, or explainability. Like how did the machine learning algorithm come to its conclusion? Why does it suggest, I don't know that this loan is, um, you know, will be given and this will not be given. This needs to be explainable and or fairness and non-discrimination or human oversight. There needs to be a human, a human in the loop, or even security. It's all part of privacy regulations already, so that's. One way we can look at it, if we look at it a little bit more concretely, and when we think back, for example, at latest reports of, of leaks of sensitive information or chat histories, um, it also underlines the need for robust privacy and security measures in, in AI. So when we, for example, um. Let's, let's take three concrete examples how privacy is, is, uh, involved or, uh, threatened, let's say, let's put it that way by, um, by machine learning or AI. So, transparency. So, uh, there's a broad consensus in general that information provided to individuals about how their data is collected and processed should both be. Accessible and sufficiently detailed to really empower individuals to exercise their rights. So, I need to know, I have the right to know which data do you have about me, I have the right to correct the data if it's wrong, and I most often have the time that you would delete the data that I, that you have about me. So, In short, organizations using AI should be able to explain how the system makes decisions and, uh, to end users, um, and, uh, and this requirement is, of course, not easy to live up to when trying to translate or anticipate, uh, algorithmic predictions. In many cases, it is not even. possible, right? Even if you wanted to explain it, you cannot. Um, that's a big, big issue. And there's this vast like research area of explainability also with various taxonomies. It's very, very complex. There's a lot of, um, effort going into this field. But I think a second issue is even if you have like explain, explainability methods such as. SHAP or LIME. Those are surrogate models explaining how the other model most likely came to specific conclusions. How do you even translate this complexity to the end user? So I have not personally found the answer to that yet. And another issue I mentioned, um, a data subject, right? So rights of individuals. One of those rights is for example, the right to be forgotten. So how do you really do that if you have. your data, if your data has been part of the training data. So while removing data from a database might be comparatively easy, it is of course. It's difficult to delete data from a machine learning model and doing so may undermine the utility of the model itself. So, uh, or a third problem that we have is in general, this, uh, you know, web scraping because large language models, as we know, are trained on a mix of, of data sets, including data scraped from the internet. And this is not regulated in the same way globally, so we have, on one side of the spectrum, U. S. state privacy regulations, such as, for example, in California, in general, uh, uh, data that is lawfully made available to the general public is out of the scope of the U. California Consumer Privacy Act. So it's not protected because it's public. Um, nevertheless, it can still create a liability risk for web scrapers when, when you do not see or are not aware that the website's terms of service explicitly prohibit. data extraction, or if you extract data or crawl data that is password protected, for example. And on the other side, in Europe, under the GDPR, you also need an explicit legal basis, even for the collection and processing of public data. So I think those are some privacy related questions that are Super challenging and hard to solve. I personally still like where like things are going in general. I love, um, a lot of services that are, you know, uh, AI and machine learning make possible. So I do hope that with this immense, uh, research areas, and so many people working enthusiastically on those problems, trying to really find solutions that we will move forward with. I don't know, differential privacy might be a solution or deduplication, or there is a machine unlearning, trying to solve problems of deleting data from a machine learning model, so or reinforcement learning with human feedback to, I don't know, um, solve the problem of, of output, uh, outputs or malicious outputs. So I do hope that, um, we do find solutions to those problems. Do not just have to shut everything down like Italy tried to do at the beginning with ChatGPT, right?
Chris Romeo:Yeah, it seems like when I think about all of the big technology moves in the last 25 years, as I've, since I've been a working professional, AI and machine learning seems like one of the ones where we're most ahead of trying to understand it, secure it, and protect the privacy of things that are happening within it. It just seems like we have, based on all the documents that you referenced as far as requirements and, and principles and things that different parties are writing, it just, it gives me hope that we're, that we're, we're, we're more ahead of the game than I've ever seen us be ahead of the game of anything. in the world of technology. And so I don't know, I mean, Katarina, what's your take on that? Do you feel like we, we're, we are ahead of the game of where we need to be? Are we behind the game when it comes to security and privacy for AI? Or are we in the right spot? What's your take as somebody who's a lot closer to this than we are?
Katharina Koerner:Um, I think it's great that the general awareness and also like, um, at least policy support, you know, by documents that are not legally binding, but still, you know, are some signal that there is awareness that this is all happening. I do not personally think that with, especially with privacy, we're ahead of the game because my impression is that a lot of services were built without having privacy. As a, um, you know, as a thought from the onset on, so with privacy by design or privacy engineering in mind, I do think though that that is likely to, that is likely to change. So when we have privacy assessments of, uh, complex, uh, LLMs, and we We'll have to come, if we will have to come to the conclusion, I mean, I don't know, cannot possibly know, that they do not meet specific privacy requirements. Then I think it's a call that the next models or next specific services, we will build in a better way so that privacy security or responsible, uh, responsibility by design, this whole shift left. will, you know, be more, um, obviously needed and done. So I recently read an article that about like, um, AI governance platform. So startups governance. There are many companies now, um, coming up with AI governance solutions, uh, which are horizontal, like all use cases, like, uh, and that article written by some VCs, I guess here in Silicon Valley said, yeah, that's nice, but. As they said that, as some of those platforms already come to the conclusion that some AI services do not comply with privacy regulation, for example, that is actually also a, um, initiator or, you know, um, Motivation for companies that have sector specific services offering, uh, you know, built with a responsive AI principles in mind. And they had this example of a platform for loans, like, you know, this machine learning models, uh, helping you to decide who should get a loan, which has a specific explainable tool or explainability feature, uh, in it, because you will not. be able to offer such services on the market, I think on the longterm, if you do not have that. So I think we might be at a turning point, uh, where with all this media coverage now and this awareness that there might be problems, it's good that we know that there are problems, that we will build better products, uh, moving forward.
Robert Hurlbut:So it's, it sounds like, um, we know quite a bit now, but we're still catching up. You know, and like I said, hopefully some turning points here, but we're still catching up with making sure that it's a much more secure and certainly attention to privacy. Let's think about a little bit about ethical use of AI. How does security play a role in ensuring that Ensuring that AI behaves predictably and ethically, as well as if you may be able to share an example where a lack of security led to unintended ethical consequences.
Katharina Koerner:So, um, I'm sure that other takes on this, um, but I think secure systems are ethical systems. And the principles are, uh, interdependent, uh, sometimes with trade offs. I mean, they see that the principal, responsible AI principles are interdependent. And sometimes, you know, you, you have to see, uh, which one is you prioritize, such as for example, transparency and security, transparency in a general way. What's, what has happened, what have you done and stuff like that. That's why we may not hear from major AI security incidents. Yeah, because I do not really categorize like deep fakes or ransomware now under AI security incidents, because it's, it's not new, right? You know, way more about this than me. What I find interesting to maybe call out here is the report published by, um, Google's AI Red team, only this July for the first time, actually, um, and just a month before in June, Google had introduced its secure AI, uh, framework to address risks, uh, to AI systems and, and drive security standards for them. And in this new report, they introduced the, their AI Red team and explained, you know, what. Red teaming is in the context of AI, why it's important, what time of attacks they simulate and lessons they've learned that they can share with others. So they said they have already revealed vulnerabilities and weaknesses that didn't go into detail, um, which ones. What I found interesting, and I think that might be something that was at least I was happy to hear that not only because it is so important, because it's recognized, because it's acknowledged, because it's, um, appreciated, but yeah, uh, but yeah, because it's just true because what they also said that, uh, while, um, that traditional and actually all sources that I came across always, they all emphasize that.... Traditional security controls like insuring systems and model security, they can already reduce risk in AI systems in a significant way. And just traditional, good, mature security programs are the basis for everything, like for everything else that might be come on top of that, you know, coming with AI and machine learning. So Google's report also said that many attacks on AI systems, they can be detected using the same methods as traditional attacks. So I think that you are. us as security, uh, professionals, or I wouldn't count myself as a security professional, but I have big appreciation for security. So I'm, I'm really glad that this is acknowledged. It's not about some fancy thing, you know, but, um, it's really about robust. Very basic, as if it wasn't, you know, enough work security, um, um, security measures or security practices of security posture. And on top of that, we then, that's also emphasized pretty often by those reports that I mentioned, then we need this really good communication between security professionals and data scientists, so that not. You know, each one of them has to become the expert in the field of the other, but we all need to learn from each other. And the same is true in general with AI. I think like, you know, legal people with all this, um, AI legal requirements being on the table or coming up, we need to talk to each other because we cannot become experts in each other's domains. So this, I think this trust or this safe environment that we can share and ask each other questions. And learn from each other and find common solutions is so super important. And this is why also in general AI governance in organizations, there's usually always either AI ethics board or just a working group on AI, where we all come to the table, like legal people, security, privacy, product, um, and so forth. And this is something that I would really like to emphasize because sometimes it can feel. Um, Would see frightening, but you know, awkward to ask like simple, seemingly simple questions. But I think we live in such a complex world that, that it's, um, there is no shame in asking. I love to ask simple questions. I love to work with engineers because I'm always, you know, I can ask the stupid questions. And usually they're, you know, a bunch of other people are also like, I'm so glad she asked the question because, um, um, yeah, so we should not be afraid of. learning from each other and really, uh, asking each other questions to better understand what's going on. Sorry that I drifted a bit, you know, away from your question, but that was somehow also on top of my mind.
Chris Romeo:No, I think it's a, it's a, it's a good reminder that it's okay to ask questions. I do the same thing. Like I, and I would say early in my career, I was always afraid to ask questions because I didn't want to seem like I didn't know the answer. And, The longer I've been doing this, the more I realize how much I don't know. And so I just ask questions because I want to learn. I want to know, I want to understand these things. And, and nobody in our industry knows everything. If anybody tells you they know everything, run away, run away from them quickly because nobody knows everything. This is so complicated. Our industry has changed. In this single day that we've all been working, our industry, there's been changes in our industry, new things have been invented, new concepts are being implemented, like, nobody knows all that stuff, that's, I just, I just can't, uh, I can't believe it. So, future of AI. What's the future of security and AI? Like, Katharina, when you think about five years down the road, what are you hoping... Exists. What are you hoping has happened so that if we get five years down the road, we're looking back and we're like, Oh yeah, these things all came together and we feel, we feel better about security and AI. What, what would that list contain for you?
Katharina Koerner:So I would first imagine that there is this role of AI security engineers or AI security or machine learning security engineers. So I'm curious actually, if there is a new role emerging. yeah, data scientists and, you know, security professional. If this is a new role that is somehow coming into existence. And I would personally hope, that AI governance in general. So all of those principles, I mean, this has to sit somewhere. It's a cross functional, uh, topic for sure, but as AI and machine learning is inherently technical. Maybe not like in its harms and outcomes and, you know, the business support that it can provide and so on, but in its risks, all of the risks, even bias and, and explainability, they also have this technical component, of course, not only, but, but for a big part, I would hope that IT or the CISO would take on this responsibility to somehow host, I mean, I don't know if I need to hope this. I mean, it's just a thought that it might be the best that, you know, that the office that somehow coordinates things sits under the CISO or an IT. So I'm not quite sure. I think under the CISO, because I'm a bit concerned that if it's too much legal driven, that. We have a similar thing as in privacy and privacy engineering, where there's still this mismatch or, you know, there's, or this, you know, the lack of communication or this challenge of communication, at least and privacy engineers in general. Yeah, okay. There's the legal person and the legal person, it's very complex, right? Engineering is very complex. We, as legal people, we, it's really hard to understand the nits and grits. of how to put those things into practice. But I think with AI and machine learning, it becomes even more evident that it's about hands on operation, operationalization of those responsible AI principles and not just, you know, they shouldn't get stuck on this policy level. So I would hope that there is. Um, a lot of educational support for, uh, security professionals or even new educations to grasp and tackle AI and machine learning, and that they will really take on the responsibility and get a lot of budget for it to, to, to, to, to support those responsible AI principles in practice and not. You know, not only like on a policy and declaration level. And of course, I think there's so much, uh, um, optimism also how AI and machine learning can help with security, right? I mean, so many tools can be, can get so much better with security. It's the same thing as in privacy. We have so much, um, transparency, uh, enhancing technologies, for example, like security tools that get somehow not repurposed, but build on those. Or as an example, like code scanning for, uh, privacy, personal, uh, information, or, uh, using APIs for communicating, uh, privacy policy. Policies of microservices, or you have a privacy taxonomy and you somehow, um, uh, code the privacy taxonomy, you know, you do privacy by code. So you really know which personal information for which purpose is where. So there's so many, um, so many opportunities also that are. Uh, on the horizon with machine learning for better security and better privacy. And I hope that this is also something that, um, companies will embrace and that they will not be only driven by enforcement because we know that enforcement generally also lacks budget. So, um, so this trust is a general principle for in our data driven economy that can be really supported by having better. using those tools that are already on the market, uh, uh, yeah, in, in more.
Chris Romeo:Yeah, that's a, that's a good sounding future though that you're describing. Uh, roles where we have AI security engineers that are, that are in a particular role focused on security and privacy of the models and, and all of the things that, that are, that are dragged in when we think about AI, um, also proper placement in the organization. was another thing that I heard you discuss there and that's from a, from a governance perspective, from an ability to influence change, from a budget perspective, those are all important things to cement a more solid and secure future for AI with security and privacy both. Okay, I think we're ready for the lightning round, where Robert takes you through a number of really quick questions. So, Robert, I'm wondering if we want to tweak this first question, because normally we'd be asking what's the most controversial, your most controversial opinion on application security, but I think after hearing all of your answers, Katarina, I really want to hear your most controversial opinion on artificial intelligence.
Robert Hurlbut:Hmm.
Chris Romeo:we get that? Can we do that substitution here? Like what's something contra, a controversial opinion that you have about artificial intelligence?
Katharina Koerner:Well, I mean, my last initiative was. is actually um, uh, AI education network. I came up with this initiative. Um, it's about educating children about AI and teachers. Uh, so here I think the controversial opinion is that I see is like Is it a bad thing? Like, will our kids get, you know, all like root? Or is it a good thing? And, um, I personally think, you know, embrace it because books, I think as, as far as I can not remember, but have read, they were also like controversial at the beginning of, oh God, my kid will get, you know, all, I don't know, lost. get lost in a fantasy world with reading books. So I think we should embrace this opportunity, for example, using creativity in schools with clear guidelines, how and so on. And, um, I, I'm currently trying to, uh, come up with this initiative to educate a little bit about more. More about this space. So the controversial opinion is, is it like spoiling and ruining kids? Or is it like, you know, something that will, will they not be able to write an essay anymore in the future? Or, or can we embrace it so that they learn how to use it properly and, you know, never, ever like stop thinking for themselves.
Robert Hurlbut:That's a great one. Uh, so this, this next question is, um, what would it say if you could display a single message on a billboard at the RSA or a Black HAT Conference or any security conference? Uh, what would be a message you'd want people to see?
Katharina Koerner:I like that question. And I would display: high expectations, high budget. Low expectations, low budget. I mean, people who go to RSA probably know that, so we should probably like have it more like out outside of Moscone center or something, but yeah, I mean, it's incredible, the budget.
Chris Romeo:Oh, that's good. That's a, that's a very, it's a, it's thought provoking. It makes you stop and think about it for a second. Like, wait, what are my expectations? Do I have an expectation of, uh, do I have low expectations? And that's why, uh, that's what my budget drives me to, though.
Robert Hurlbut:So the third one is what's your top book recommendation and why do you find it? Valuable.
Katharina Koerner:So Thank you. I really liked the book The Ethical Algorithm. It's by Michael Kearns and Aaron Roth. It's about algorithmic design. It sounds Thanks. Again, like I find ethical personally a bit fluffy, but it's like really hands on and breaking down like for, you know, readers who are not like machine learning experts, uh, how to design algorithms that are privacy, uh, preserving, uh, that are, how, what's the problem with, um, non bias, uh, computational non bias. And so. in a very, um, clear and somewhat engaging style. So I really recommend this book, although it sounds, you know, a bit abstract, but actually it's super concrete and it's a really, really good book. The Ethical Algorithm.
Robert Hurlbut:Pretty cool.
Chris Romeo:Excellent. That's, that'll be one to check out. I, I love that we asked this question because it keeps filling up my list of books to read. Things that are outside, and this one's not one that I would normally have even gone looking for, but that sounds really fascinating and I want to understand. I want to understand what, uh, what I can learn there. So, Katharina, what, um, what's kind of a key takeaway as we, as we wrap up our conversation? Maybe a call to action. Is there something that you want our audience to do? as a result of our conversation today.
Katharina Koerner:So I would recommend, and I'm recommending this to myself again. I mean, of course I have had a look already, but I will, I need to like. Read this again and again. I think we should familiarize ourselves, especially as we're based in the U. S. with the NIST AI Risk Management Framework because I think while the EU is coming up with this It's still a draft, but they will pass the EU AI Act and the EU AI Act will require, uh, risk management frameworks for high risk, uh, machine learning and AI systems. I think in the US where we do not have, uh, you know, uh, comprehensive AI law on the federal level, I think the US will push the NIST AI Risk Management Framework as their like. Um, uh, you know, global contribution to, uh, AI governance and nist uh, is also, I mean, they always to that, that have, they will also develop those crosswalks to, of course also the cybersecurity risk management framework. And I think if we. Have at least had a look at it, you know, um, aware that there's a NIST AI Risk Management Framework that is just, um, it positions us as subject matter experts in a very good way that we are part of the current, um, This course and conversation and that we're aware of what's going on. And then, you know, maybe we can also already develop some thoughts. I mean, NIST is always, I find it always a bit hard. It's not super accessible, but in the end, and it's also, you know, it's also, um, all about those responsible AI. So of course, security is one principle. So, and when the point will come, the point in time will come where we're like supposed to merge, uh, security risk management frameworks that we already use, and maybe, you know, we have some, took some inspiration or even have implemented everything according to NIST, then we can already contribute to some conversation that how this relates to the NIST AI Risk Management Framework or how both resources, or especially the new one, can contribute to our organization's risk management framework. So I think that would put us personally and the organization in a good starting position, uh, moving forward.
Chris Romeo:Excellent. Well, I'm gonna, I'm gonna go take a closer look at that. I have not looked at the NIST AI Risk Management Framework. I've looked at many other risk management frameworks that NIST has created over the years. But, uh, I'm gonna go check that one out as well. So, Katharina, thank you for being with us today, sharing your knowledge and experience about AI and security and privacy. Um, this has been excellent. I've learned a lot in this conversation. And now... Now I've got to go study some more, get deeper into these things because you, you piqued my interest in a number of different pieces about, uh, the ethical side of AI and how security and privacy fit in together. So thank you very much and thanks for being with us.
Katharina Koerner:Thank you very much for having me.